讲座时间:2025年04月21日(星期一)下午15:50
讲座地点:犀浦校区3号教学楼X31541报告厅
主讲人:Xiatian Zhu教授
主讲人简介:
Dr. Xiatian Zhu is a Senior Lecturer at the Surrey Institute of People-Centred AI and the Centre for Vision, Speech, and Signal Processing (CVSSP) at the University of Surrey in Guildford, UK. He leads the Universal Perception (UP) lab, which focuses on advancing multimodal generative AI for real-world applications and business. Dr. Zhu earned his PhD from Queen Mary University of London and received the 2016 Sullivan Doctoral Thesis Prize from the British Machine Vision Association. His contributions include the development and commercialization of multi-camera object association systems for industry. His work has garnered several best paper awards, and he has been recognized as one of the UK's and the world's best rising stars in science. Dr. Zhu's extensive research output includes over 150 articles in top-tier conferences and journals, with more than 20,000 citations and an H-index of 58. Additionally, Dr. Zhu holds five US patents in the fields of computer vision and AI.
内容简介:
AI models often struggle when deployed in environments that differ from those they were originally trained on. For example, an image recognition system trained on clip art photos may perform poorly on painting images. Adapting AI models to new domains is critical for reliable performance, yet direct access to the original training data is frequently restricted due to privacy, legal, or logistical constraints. In this talk, we explore how AI systems can still be adapted using only unlabelled data from the target domain and noisy signals from external sources, such as large vision-language models. We present a denoising-based method that refines this noisy guidance and aligns it with the target data, enabling more robust and flexible AI adaptation across varied scenarios. Our results demonstrate strong performance, offering a practical solution for deploying adaptable AI in real-world, data-constrained settings.
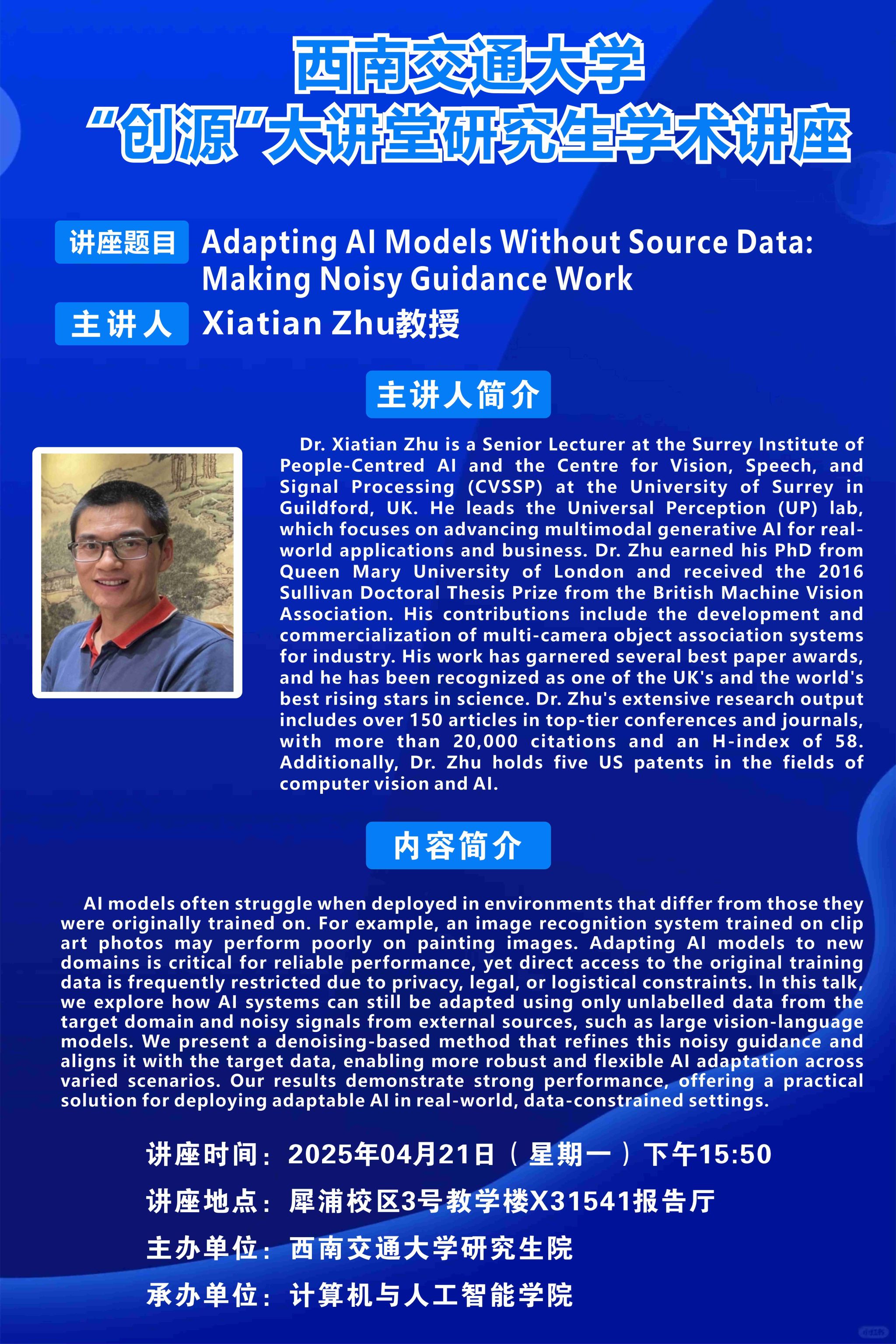