讲座时间:2023年12月11日(星期一)上午10:00
讲座地点:犀浦校区X31541学术报告厅
主讲人:任钊副教授
主讲人简介:
Dr. Zhao Ren is an Associate Professor in the Department of Statistics at the University of Pittsburgh. Dr. Ren obtained his Ph.D. in Statistics at Yale University in 2014. His research interests are in high-dimensional statistical inference, covariance/precision matrix estimation, robust inference, graphical models, nonparametric function estimation, and applications in statistical genomics.
讲座题目:Heteroskedastic Sparse PCA in High Dimensions
内容简介:
Principal component analysis(PCA) is one of the most commonly used techniques for dimension reduction and feature extraction. Though it has been well-studied for high-dimensional sparse PCA, little is known when the noise is heteroskedastic, which turns out to be ubiquitous in many scenarios, like biological sequencing data and information network data. We propose an iterative algorithm for sparse PCA in the presence of heteroskedastic noise, which alternatively updates the estimates of the sparse eigenvectors using the power method with adaptive thresholding in one step, and imputes the diagonal values of the sample covariance matrix to reduce the estimation bias due to heteroskedasticity in the other step. Our procedure is computationally fast and provably optimal under the generalized spiked covariance model, assuming the leading eigenvectors are sparse. A comprehensive simulation study demonstrates its robustness and effectiveness in various settings.
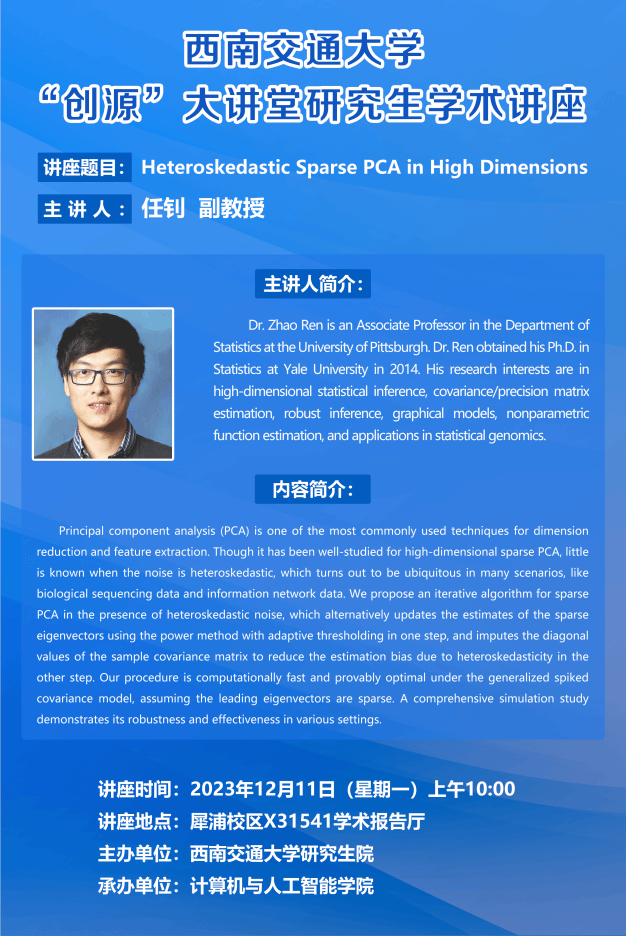