讲座时间:2024年11月25日(星期一)上午10:30
讲座地点:犀浦校区3号教学楼X31541报告厅
主讲人:德国哈根大学Herwig Unger教授
主讲人简介:
Prof. Dr.-Ing. habil. Dr. h.c. Herwig Unger (1966) received his PhD with a work on Petri Net transformation in 1994 from the Ilmenau University of Technology and his doctorate (habilitation) with a work on a fully decentralized web operating systems from the University of Rostock in 2000. Since 2006, he is a full professor at the FernUniversität in Hagen and the head of the Department of Communication Networks. In 2019, he obtained a honorary PhD in Information Technology from the King Mongkut’s University of Technology in North Bangkok (Thailand). His research interests are in decentralized systems and self-organization, natural language processing, Big Data as well as large- scale simulations. He has authored more than 150 publications in refereed journals and conferences, published or edited more than 30 books and gave over 35 invited talks and lectures in 12 countries. Besides various industrial co-operations, e.g. with Airbus Industries, he has been a guest researcher/professor at the ICSI Berkeley (USA), the University of Leipzig and other universities in Canada, Mexico and Thailand.
内容简介:
This talk presents a concise introduction to Markov Chains and processes, setting the stage for an in-depth exploration of the foundational structures and functional learning principles of the brain. Drawing on the pioneering ideas of Jeff Hawkins, it will illustrate how the human brain functions as a predictive engine, relying on advanced sequence learning and recognition mechanisms to interpret and engage with the world.
Building on these insights, the 'GraphLearner' model is introduced, designed to encapsulate some of those principles and foster an explainable learning mechanism inspired by the brain's functionality. The GraphLearner operates on the premise that understanding and processing data in a manner akin to human cognition can lead to enhanced outcomes in machine learning.
Initial experimental results will demonstrate the GraphLearner's effectiveness, particularly in the domain of Natural Language Processing (NLP). These results indicate significant improvements in comprehension and response accuracy, highlighting how this model adapts and learns from complex linguistic structures.
Furthermore, the presentation will explore the GraphLearner's capabilities in enhancing attention mechanisms, enabling parallel processing, and facilitating the formation of hierarchies. It will be shown that the GraphLearner improves machine learning efficiency across various applications while providing a framework for creating more interpretable and reliable AI systems.
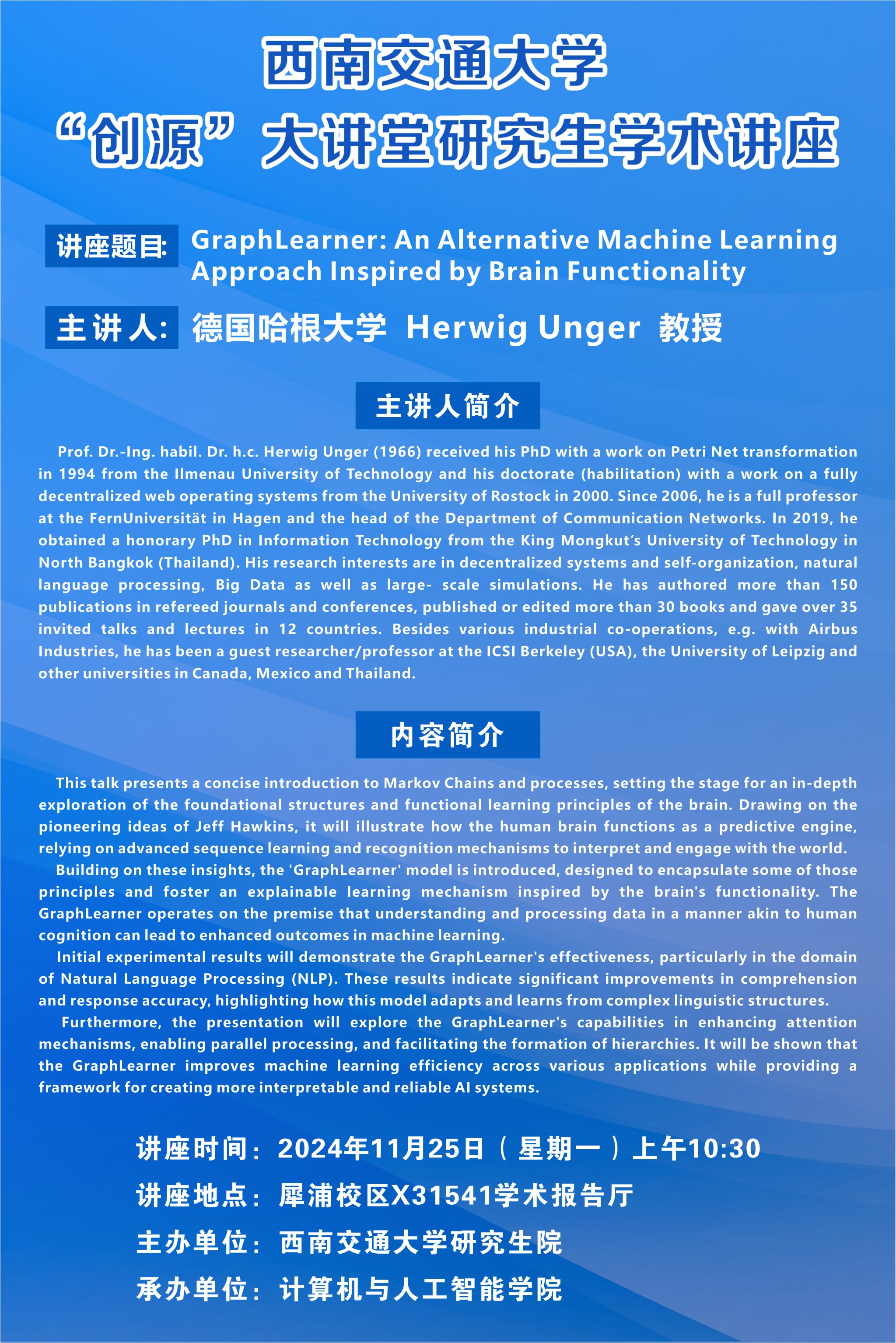